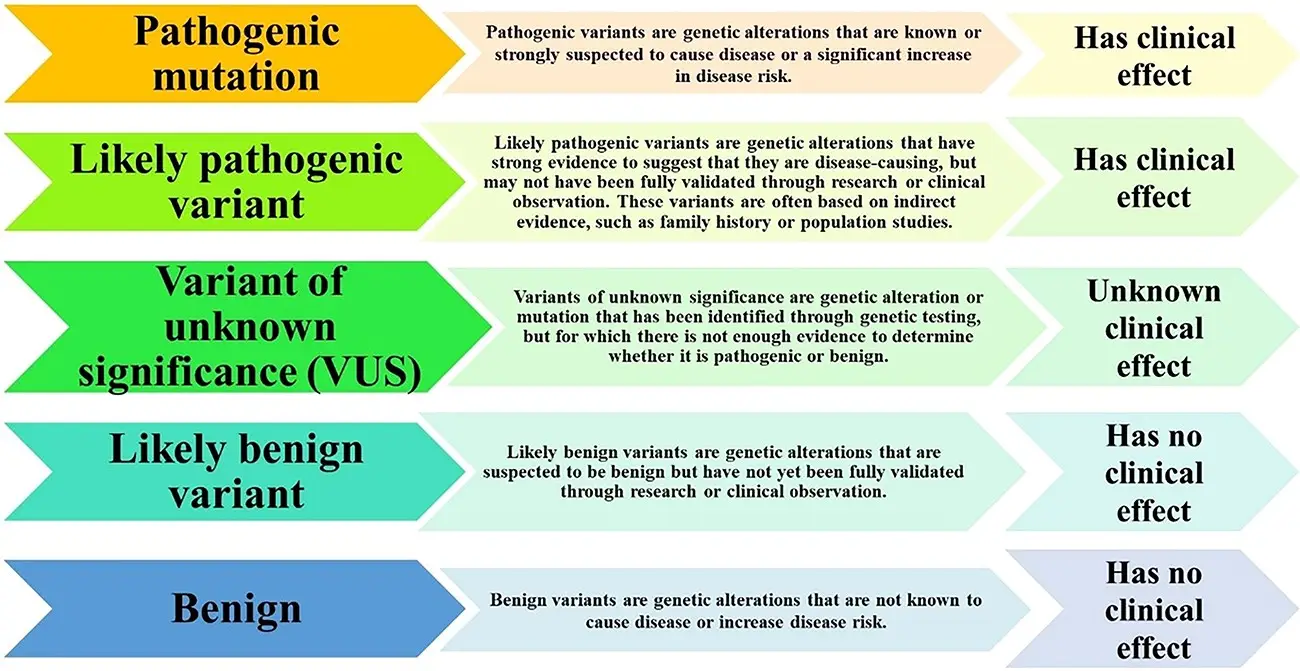
Machine Learning and Genetic Databases for Breast Cancer Pathogenicity Prediction
đź§ Introduction
As breast cancer remains one of the leading causes of cancer-related deaths worldwide, early detection through genetic insights has become increasingly vital. A new review published in Briefings in Bioinformatics explores how machine learning tools, paired with curated genetic variant databases, can better predict the pathogenicity of breast cancer-associated mutations.
🔍 Research Summary
The study presents a comprehensive evaluation of the landscape of genetic variant databases and machine learning algorithms tailored for breast cancer (BC) pathogenicity prediction. It analyzes the variety of existing tools and databases—including their design, use cases, and the types of data they rely on—to uncover inconsistencies in prediction outcomes. A key focus of the review is determining which tools are best suited for clinical and research contexts, based on their training data and performance specificity.
The authors conclude that tools trained on multiple, diverse datasets—especially those drawn from disease-specific databases—offer improved accuracy in identifying pathogenic variants. These tools are also more clinically valuable, potentially accelerating diagnosis and treatment planning for individuals at risk of breast cancer.
🌍 Broader Impact
This research aligns closely with APMAD’s Cancer theme and its broader mission to advance precision medicine in the UAE and globally. By identifying the strengths and limitations of current predictive models, the review highlights an important path forward: investing in integrative platforms that combine high-quality, disease-focused data with advanced analytics.
The findings reinforce the need for cross-disciplinary collaborations between geneticists, data scientists, and clinicians to develop more reliable, transparent, and actionable prediction tools. Such innovation will not only enhance early detection and targeted care but also support public health efforts in cancer prevention and genetic screening.
📎 Reference
Rahaf M Ahmad, Bassam R Ali, Fatma Al-Jasmi, Richard O Sinnott, Noura Al Dhaheri, Mohd Saberi Mohamad, A review of genetic variant databases and machine learning tools for predicting the pathogenicity of breast cancer, Briefings in Bioinformatics, Volume 25, Issue 1, January 2024, bbad479, https://doi.org/10.1093/bib/bbad479
Leave a Reply