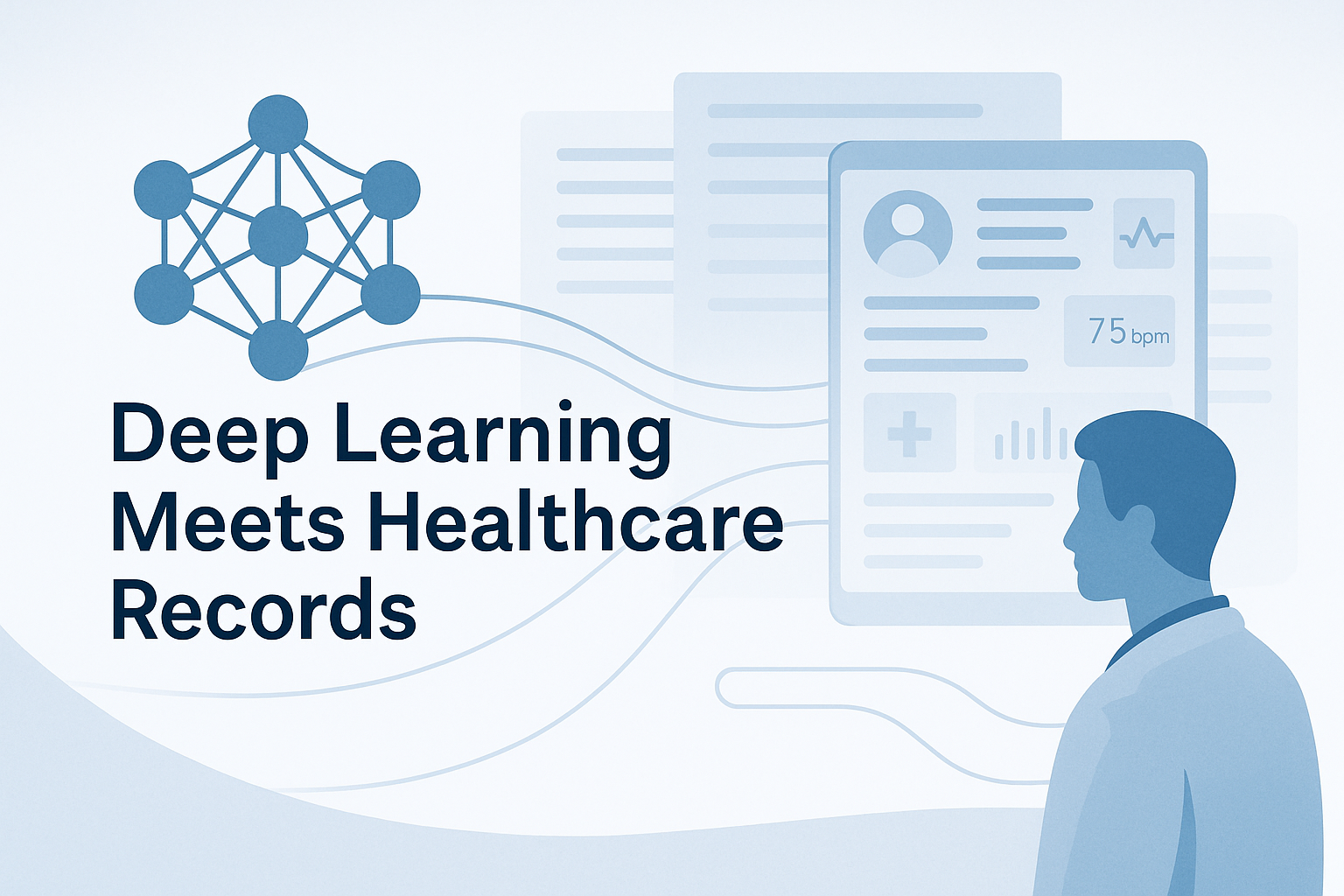
Deep Learning Models for Health Prediction: A Review of EHR-Based Approaches
🧠 Introduction
As healthcare continues its digital transformation, one challenge remains critical: how can we extract actionable insights from the vast, complex data stored in electronic health records (EHRs)? A recent review published in Artificial Intelligence Review offers a timely analysis of how deep learning models are helping researchers harness EHR data for health prediction—and what hurdles remain.
🔍 Research Summary
This paper reviews the growing use of deep learning in analyzing EHR data to forecast disease outcomes and improve diagnostic accuracy. EHRs encompass a wealth of patient information—including clinical notes, diagnoses, and demographic data—which makes them ideal for training predictive algorithms. However, their high dimensionality, heterogeneity, and limited public availability pose significant challenges.
The review synthesizes state-of-the-art deep learning architectures and the online databases currently available for EHR-based research. It categorizes models based on usability and features, aiming to help researchers choose the most effective tools for their objectives. The paper also highlights how end-to-end learning models have become increasingly viable, thanks to recent advances in AI.
🌍 Broader Impact
This study directly supports APMAD’s mission to advance data-driven precision medicine across domains such as brain health, genetic disorders, and metabolic diseases. By clarifying the landscape of EHR-based deep learning, the review empowers researchers to build more robust models—ultimately contributing to smarter clinical decision-making and improved patient outcomes.
It also underscores the need for greater access to high-quality EHR datasets, a critical bottleneck for innovation in healthcare AI. Addressing this could catalyze broader adoption of predictive tools in real-world clinical settings.
📎 Reference
Nasarudin, N.A., Al Jasmi, F., Sinnott, R.O. et al. A review of deep learning models and online healthcare databases for electronic health records and their use for health prediction. Artif Intell Rev 57, 249 (2024). https://doi.org/10.1007/s10462-024-10876-2
Leave a Reply